Stichprobenplanung für den WMW-Test
Die Formeln zur Stichprobenplanung für den Wilcoxon-Mann-Whitney Test, wie beim Oberseminar am 14.1.2015 vorgestellt, sind hier einmal in R implementiert:
brunnerWMW.R
# brunnerWMW (), an R function to estimate sample sizes for
# Wilcoxon-Mann-Whitney test based on the placement method
# due to Edgar Brunner.
#
# Copyright (C) 2015 Christian Roever
#
# This program is free software: you can redistribute it and/or modify
# it under the terms of the GNU General Public License as published by
# the Free Software Foundation, either version 3 of the License, or
# (at your option) any later version.
#
# This program is distributed in the hope that it will be useful,
# but WITHOUT ANY WARRANTY; without even the implied warranty of
# MERCHANTABILITY or FITNESS FOR A PARTICULAR PURPOSE. See the
# GNU General Public License for more details.
#
# You should have received a copy of the GNU General Public License
# along with this program. If not, see <http://www.gnu.org/licenses/>.
#
brunnerWMW <- function (x1, x2, alpha=0.05, beta=0.80, t=0.5)
# Sample size estimation for Wilcoxon-Mann-Whitney test (WMW-test)
# based on placement method due to Edgar Brunner.
#
# arguments:
# ----------
# x0, x1 : representative first and second "samples"
# alpha : level of WMW test
# beta : aimed for power
# t : proportion of samples in first sample
#
# value:
# ------
# a list containing three elements:
# – a vector containing the rounded total sample size (N),
# and the two individual sample sizes (n1, n2),
# – a vector containing the un-rounded approximate figures,
# – the estimated relative effect size (pstar).
{
# check whether input argments look sensible:
stopifnot (all(is.finite (x1)), all(is.finite(x2)),
alpha>0, alpha<1, beta>0, beta<1, t>0, t<1)
# "sample sizes":
m1 <- length (x1)
m2 <- length (x2)
# ranks among union of samples:
R <- rank (c (x1,x2), ties.method="average")
R1 <- R[1:m1]
R2 <- R[m1+(1:m2)]
# ranks within samples:
R11 <- rank (x1, ties.method="average")
R22 <- rank (x2, ties.method="average")
# placements:
P1 <- R1 - R11
P2 <- R2 - R22
# effect size:
pStar <- (mean (R2) -mean (R1)) / (m1+m2) + 0.5
# variances:
sigmaStar <- sqrt (sum ((R11-((m1+1)/2))^2) / m1^3)
sigma1Star <- sqrt (sum ((P1-mean (P1))^2) / (m1*m2^2))
sigma2Star <- sqrt (sum ((P2-mean (P2))^2) / (m1^2*m2))
# estimated sample size:
N <- (sigmaStar*qnorm (1-alpha/2) + qnorm(beta) *
sqrt (t*sigma2Star^2 + (1-t)*sigma1Star^2))^2 / (t*(1-t)*(pStar-0.5)^2)
n1 <- N*t
n2 <- N*(1-t)
return (list ("rounded"=c ("N"=ceiling (n1)
+ceiling(n2), "n1"=ceiling(n1), "n2"=ceiling(n2)),
"approximate"=c ("N"=N, "n1"=n1, "n2"=n2),
"relative.effect"=c ("pstar"=pStar)))
}
Kontakt
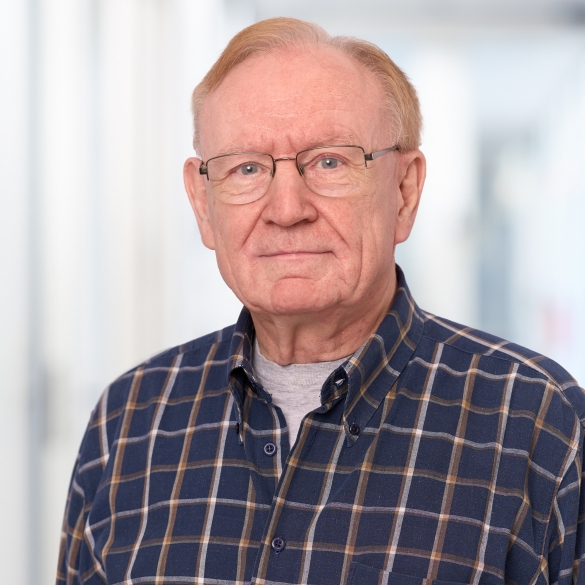
Kontaktinformationen
- Telefon: +49 551 3965028
- Telefax: +49 551 3965605
- E-Mail-Adresse: ebrunne1(at)gwdg.de
- Ort / Raum: Humboldtallee 32, EG, 115